Each year in Stockholm, Nobel Prize Week celebrates the ideas and breakthroughs that have transformed our understanding of the world. In the same week (6–12 December), the Scien...
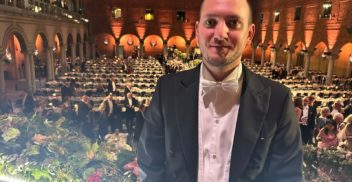
Celebrating Breakthroughs: Dr Andrija Sente Honoured During Nobel...
on Dec 16, 2024 | 10:27am