While routing a single train from point A to point B is easy, the problem intensifies when you are faced with trains breaking down randomly or how to avoid trains blocking each other at intersections. The NeurIPS 2020 Flatland challenge aims to solve this hard problem using an approach that was developed and evaluated in InstaDeep’s collaboration with Deutsche Bahn.
Since late summer 2019, InstaDeep has been working with the public German railway company on optimising and managing the dispatching and scheduling of their train network across the country using multi-agent Reinforcement Learning. In the context of the project, the Centralized Critic PPO (CCPPO) was validated and directly compared to standard PPO on a previous version of Flatland. With highly successful outcomes from the ongoing collaboration, the two companies offered the organiser SBB, who is also the creator of Flatland, to provide the baselines for the challenge.
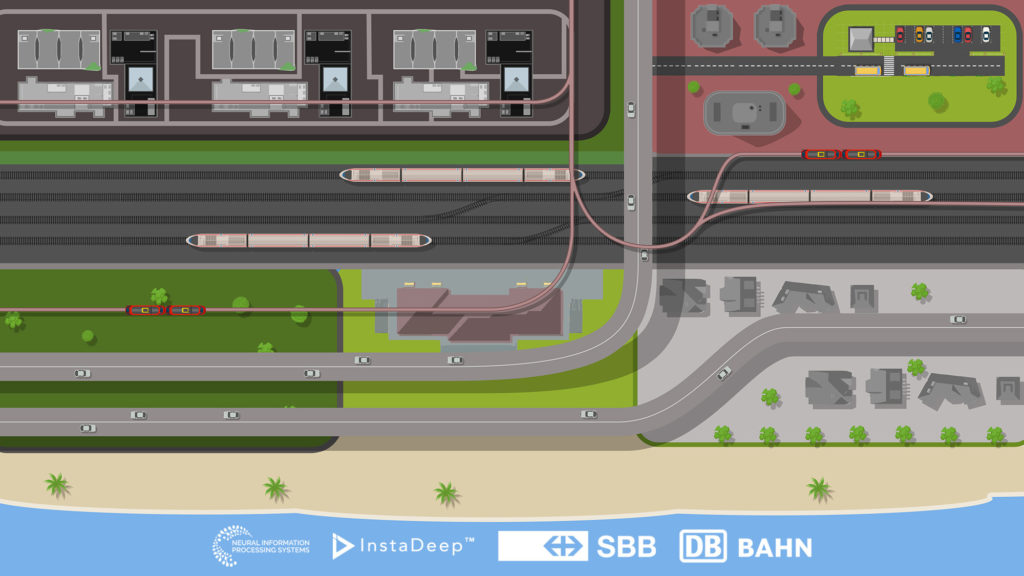
It is a great honour for InstaDeep to contribute to the Flatland challenge at the conference which is not only the world’s largest AI conference but also the industry’s most renowned. The contribution follows two successful years of NeurIPS participation. In 2018 we showcased two research papers and InstaDeep’s CEO and Co-Founder, Karim Beguir, also presented in the workshop ‘Black in AI’. In 2019, we were back, this time on the main stage presenting joint InstaDeep-Google DeepMind research. In 2020, we are proud to extend our contribution to include materials that can help drive innovation in the ML and RL space and we look forward to seeing the results that come from this challenge!
The competition is open to any methodological approach however it highly encourages using reinforcement learning as it is commonly known that operations research methods do not scale to large problem instances.
All details on how to enter can be found on the website. Further details on CCPPO, implementation details and InstaDeep’s results with DB can be found here.