The International Conference on Machine Learning (ICML) stands as one of the premier gatherings, bringing together the brightest minds from academia, industry, and research institutions. The 2024 edition is set to start in a few days in Vienna and as always our team is gearing up to attend with 7 accepted papers and 2 spotlight awards at the “Efficient and Accessible Foundation Models for Biological Discovery” and the “AI for science” workshops.
This year’s line-up of research topics showcase our continued commitment to AI innovation and demonstrate our unwavering dedication to address real-world challenges in different fields.
Enhancing the truthfulness of LLMs
Recent advancements in large language models (LLMs) underscore their potential for responding to inquiries in various domains. However, ensuring that generative agents provide accurate and reliable answers remains an ongoing challenge.
In this context, our Main track paper “Should we be going MAD? A Look at Multi-Agent Debate Strategies for LLMs” investigates promising multi-agent debate strategies for enhancing the truthfulness of LLM agents. This study benchmarks various debating and prompting strategies, finding that while MAD systems do not always outperform others, hyperparameter tuning can significantly enhance their performance. We build on these results to offer insights into improving debating strategies, such as adjusting agent agreement levels, which can significantly enhance performance and even surpass other non-debate protocols evaluated. We provide an open-source repository to the community with several state-of-the-art protocols together with evaluation scripts to benchmark across popular research datasets.
- Full paper link: https://openreview.net/pdf?id=CrUmgUaAQp
- Code link: https://github.com/instadeepai/DebateLLM
- Poster: #2208, Hall C 4-9 / Thu 25 Jul 1:30 p.m — 3 p.m GMT+2
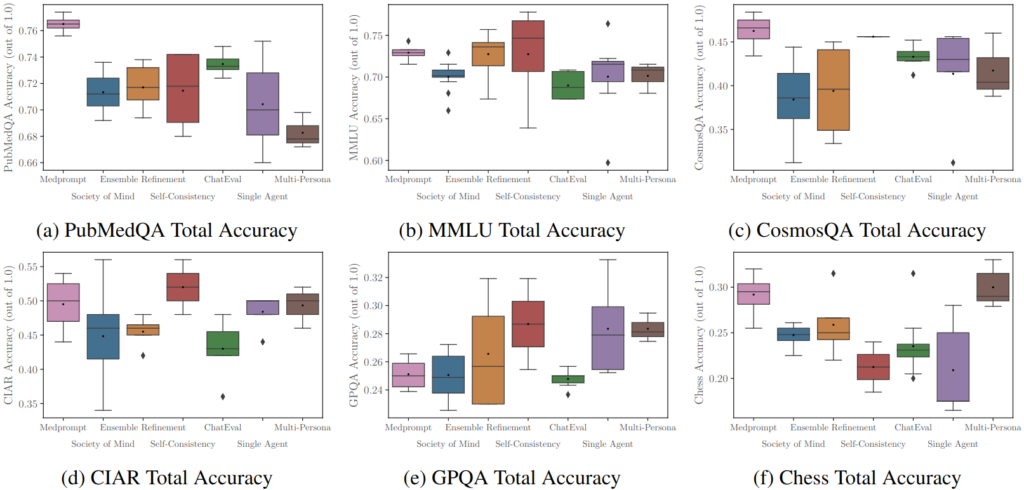
variability and robustness of each system’s performance.
Explore our Workshop Publications:
Overconfident Oracles: Limitations of In Silico Sequence Design Benchmarking
We highlight critical limitations of the current in silico protein and DNA sequence design benchmarks, and further, introduce additional biophysical measures to improve the robustness and reliability of in silico design methods.
- Full paper link: https://openreview.net/forum?id=fPBCnJKXUb
- Workshops:
- Women in Machine Learning: Poster presentation, Mon 22 Jul , 4 – 5.30pm GMT+2
- AI for Science: Spotlight, Fri 26 Jul
SMX: Sequential Monte Carlo Planning for Expert Iteration
Introducing SMX, a model-based planning algorithm using Sequential Monte Carlo methods as a scalable sample-based search. SMX demonstrates a statistically significant improvement in performance exceeding AlphaZero and model-free methods across both continuous and discrete environments.
- Full paper link: https://openreview.net/forum?id=a5hWhriatS
- Workshop: Foundation of RL and control, Sat 27 Jul
Generative Model for Small Molecules with Latent Space RL Fine-Tuning to Protein Targets
We propose a latent-variable transformer model for small molecules trained with a variant of the SAFE representation called SAFER. Using SAFER, we significantly increase the percentage of valid and chemically stable molecules with a validity rate above 90% and a fragmentation rate less than 1%. Furthermore, fine tuning the model with Reinforcement Learning improves molecular docking for five protein targets, nearly doubling hit candidates for certain targets and achieving top 5% mean docking scores comparable to or better than current state-of-the-art methods.
- Full paper link: https://openreview.net/pdf?id=OwXODhPdLt
- Workshop: AccML.Bio, Poster #35929, Sat 27 Jul
Quality-Diversity for One-Shot Biological Sequence Design
We introduce a Quality-Diversity method based on Map-Elites algorithm to conservatively score biological sequences using only a limited dataset, along with a novel way to represent biological sequences in a low dimensional way to facilitate the illumination procedure. We show our methodology to consistently improve over both traditional and novel tailored methods on 3 datasets.
- Full paper link https://openreview.net/forum?id=ZZPwFG5W7o
- Workshop: ML4LMS, Fri 26 Jul
Likelihood-based fine-tuning of protein language models for few-shot fitness prediction and design
We extend previously proposed ranking-based loss functions to adapt the likelihoods of family-based and masked protein language models, and demonstrate that the best configurations outperform state-of-the-art approaches in both the few-shot fine-tuning and multi-round design settings.
- Full paper link: https://openreview.net/pdf?id=MkYhOEUJyi
- Workshops:
- AccML.Bio: Spotlight talk, Sat 27 July 9:40 a.m GMT+2
- ML4LMS: Poster session, Fri 26 July
Coordination Failure in Cooperative Offline MARL
We use polynomial games to demonstrate coordination failure in offline MARL, and propose a solution using prioritised replay. The paper shows how insights drawn from simplified, tractable games can lead to useful, theoretically grounded insights that transfer to more complex contexts. A core dimension of offering is an interactive notebook, from which almost all of our results can be reproduced, in a browser.
- Full paper link: https://arxiv.org/abs/2407.01343
- Code link: https://tinyurl.com/pjap-polygames
- Workshop: ARLET Workshop, Fri 26 Jul
If you’re at ICML, be sure to visit our posters and join our team to explore our different research areas and to learn more about the exciting projects we are working on.
Check our research page for more information and our open opportunities at www.instadeep.com/careers.