DNA is the blueprint of life, the universal code of A, T, C, and G that guides the functioning of all living organisms, from humans to bacteria. Packed into the genome, this massive instruction manual varies in complexity across species and contains billions of letters that determine traits, health, and evolution. Small variations in DNA can profoundly affect health or responses to medicines, making genomic understanding essential for advancing medicine. However, studying this vast and intricate code is challenging due to its size, complexity, and the cost of traditional experiments.
In our paper, published in Nature Methods, we are proud to share Nucleotide Transformers (NT), a family of Foundation Models trained on a giant corpus of human and multispecies genomics data. Nucleotide Transformer marks a significant milestone in the integration of our latest innovation in machine learning and genomics and redefines our approach to genomic prediction tasks, enabling improved performance over specialized methods across a diverse range of applications.
Transforming Genomics with Foundation Models
Drawing inspiration from natural language processing, we adapted the Transformer architecture to learn the complex patterns within DNA sequences. Spanning parameter counts from 50 million to 2.5 billion, the NT family incorporates genomic information from 3,202 human genomes and 850 species, representing one of the most diverse datasets used to train models for genomics to date.
By training on this scale, the NT models achieve context-specific nucleotide representations, offering significantly increased accuracy in low-data settings. This capability facilitates tasks ranging from splice site prediction to enhancer activity modeling, underscoring NT’s versatility as a platform for molecular phenotype prediction.
Our models underwent rigorous evaluation, including cross-validation and benchmarking against state-of-the-art foundation model competitors such as DNABERT and Enformer, and task-specialized models. The results are clear: when fine-tuned, the Nucleotide Transformer not only matches, but often exceeds, the capabilities of these models, from histone modification to transcription factor binding site prediction. Importantly, we demonstrated that the integration of genomes from diverse species is key to improving our understanding of the human genome.
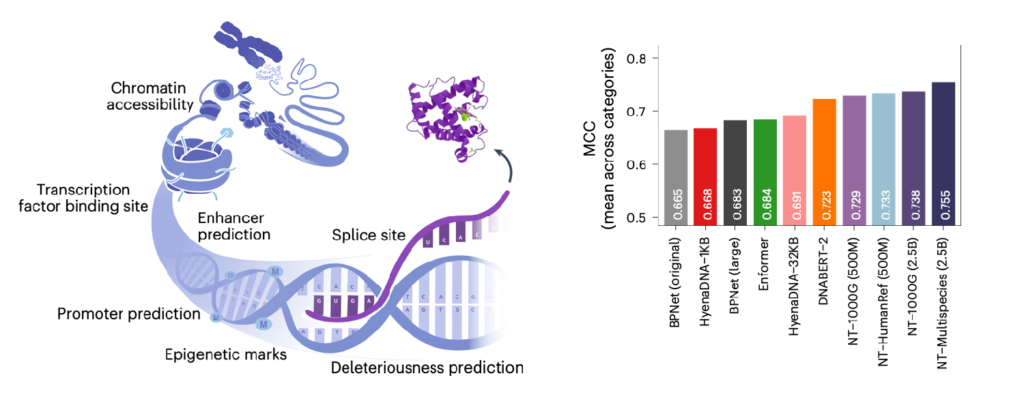
The Nucleotide Transformer opens doors to novel applications in genomics. Intriguingly, even probing of intermediate layers reveals rich contextual embeddings that capture key genomic features, such as promoters and enhancers, despite no supervision during training. In the paper, authors show that the zero-shot learning capabilities of NT enable predict the impact of genetic mutations, offering potentially new tools for understanding disease mechanisms.
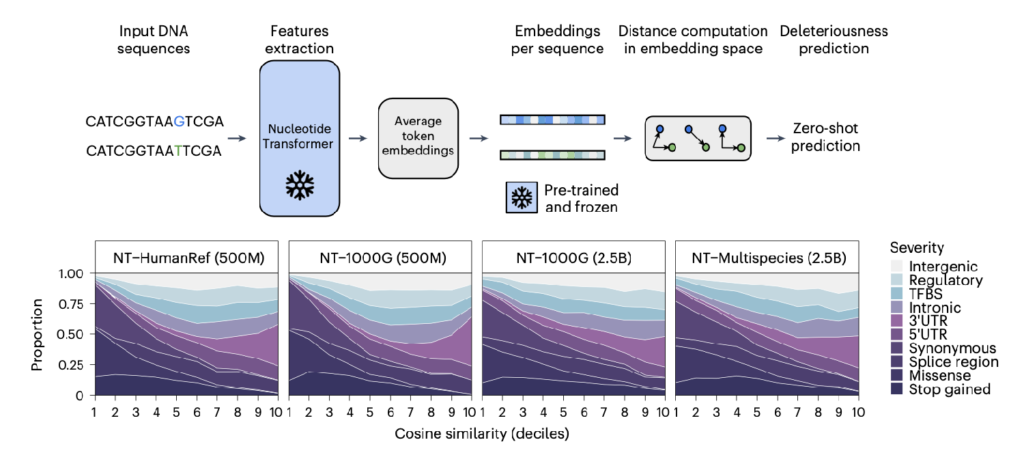
Open Science: NT Models Released
InstaDeep’s commitment to open science continues. We made the Nucleotide Transformer models and benchmarks available on the Hugging Face platforms where they have been already downloaded more than 700,000 times, with 120+ citations, illustrating the depth of interest and relevance of the work for the scientific research community. We invite other researchers worldwide to explore the potential of NT in their own work. These models are also commercially available through DeepChain, our Life Science platform serving our foundation models in biology.
This achievement would not have been possible without the collaboration of world-class institutions and cutting-edge technology. NVIDIA’s Cambridge-1 supercomputer played a pivotal role, providing compute resources needed to train the models. Additionally, the Technical University of Munich (TUM) provided crucial research support, helping refine the methodologies and validate findings.
With the rapidly expanding landscape of multi-omics data, we anticipate that these models will serve as a foundation for further breakthroughs in personalized medicine, evolutionary biology, and beyond. By bridging AI and genomics, we take a step closer to decoding our DNA.
Read more about Nucleotide Transformer in our open access Nature Methods paper, in the Nature Methods research briefing and access the models on Hugging Face or directly through Github. Want to get started? Check out our notebook tutorials.
NOTE: All Nucleotide Transformer statistics and findings in this blog are sourced from the research paper, available on bioRxiv.