As our fifth member of the fast-growing team in The Mother City, Kale-ab is already well-known in the AI industry and community! In addition to being a highly-skilled research engineer, he has an impressive track record of doing AI for good including being a dedicated member of Deep Learning Indaba (DLI), an active contributor to… Read more »
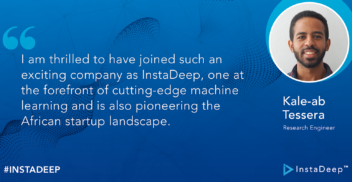